A/B Testing, Confidence Interval, P-Value
- 1. A/B Test Example
- 2. 자세한 설명
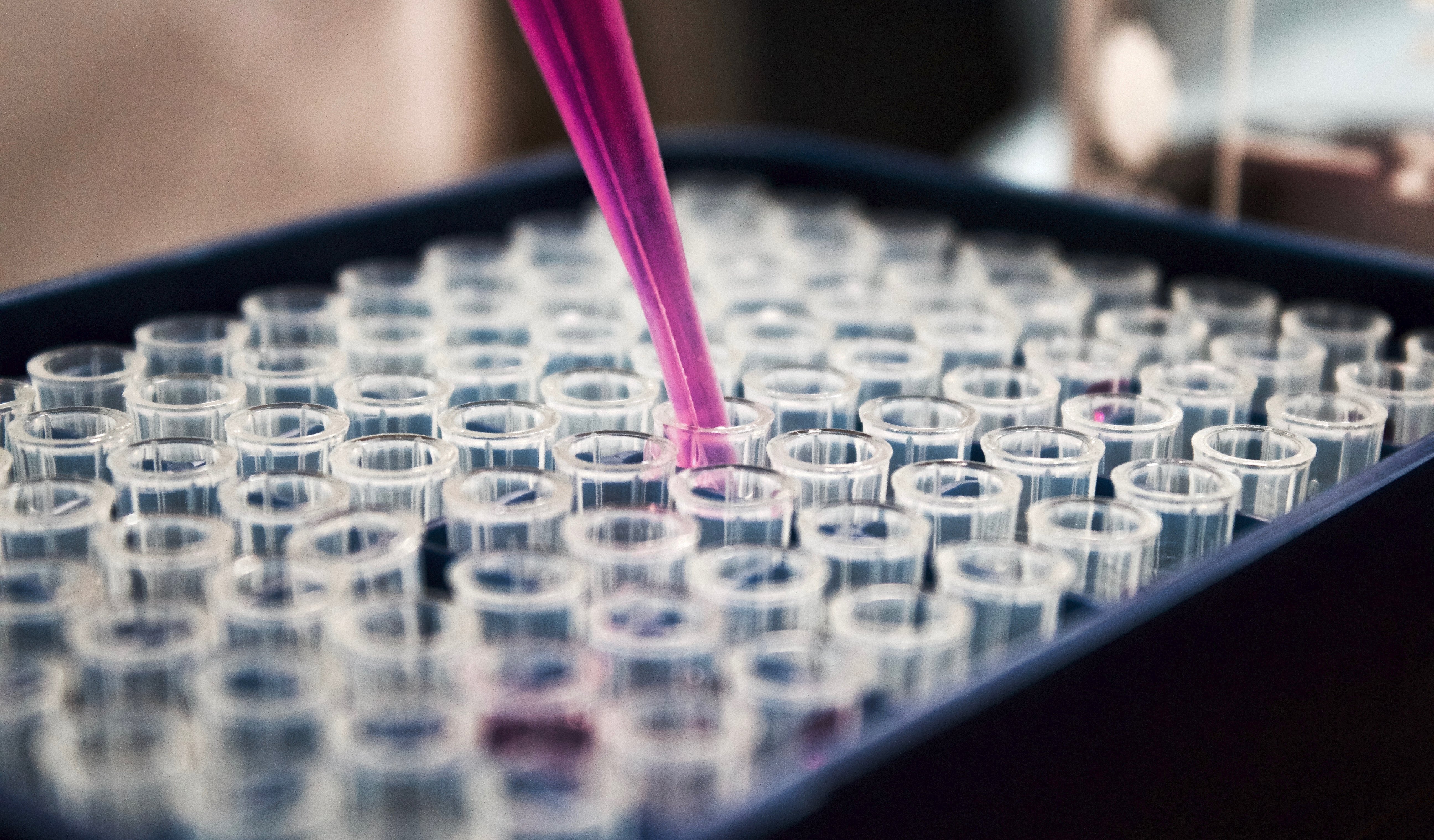
1. A/B Test Example
Variation | Conversion | View | Conversion Rate | SE | Confidence Interval | Uplift | Confidence |
---|---|---|---|---|---|---|---|
Variation A (Control Group) | 330 | 1093 | 30.19% | 0.013886 | \(\pm 2.722\) % | -11.93% | - |
Variation B (Test Group) | 385 | 1123 | 34.28% | 0.014164 | \(\pm 2.766\) % | 13.55% |
- SE: Standard Error
- Confidence Level: 95%
- conversion rate lift (Uplift): 13.55%
- Z-Score: 2.06246
- P-Value: 0.01958
1.1 Conversion Rate (전환율)
각각의 variation마다 conversion rate를 다음과 같이 계산 할 수 있습니다.
\[\text{conversion rate} = p = \frac{\text{event count}}{\text{view count}}\]위의 A/B Text를 예로 든다면..
\[\begin{align} p_a &= \frac{330}{1093} = 0.3019213174748399 \\ p_b &= \frac{385}{1123} = 0.3428317008014248 \end{align}\]1.2 Relative Lift (Relative Change)
Variation A 그리고 variation B가 얼마나 변화 되었는지는 다음의 공식으로 계산 합니다.
\[\begin{align} \text{Relative Lift}_{B} &= \frac{p_b - p_a}{p_a} = \frac{p_b}{p_a} - 1 \\ &= \frac{0.34283}{0.30192} -1 = 0.13550014841199168 \end{align}\]즉 variant B는 variant A에 비해서 13.55%의 전환율 향상이 더 있습니다.
1.3 Standard Error
The standard deviation of the sample mean 을 standard error라고 합니다.
쉽게 말해서 population을 말할때는 standard deviation이라고 말하고, sample에 관해서 말할때는 standard error 를 사용합니다.
이때 A/B테스트에서는 yes 또는 no처럼 2가지 경우만 있기 때문에 베르누이 분포를 따른다고 가정을 하며,
베르누이 분포의 standard deviation은 \(\sigma = \sqrt{p(1-p)}\) 입니다.
- \(p\) : conversion rate
- \(n\) : sample size (view)
위의 예를 든다면 다음과 같습니다.
\[\begin{align} SE_{a} &= \sqrt{\frac{0.3019 * (1-0.3019)}{1093}} = 0.013886378416134985 \\ SE_{b} &= \sqrt{\frac{0.3428 * (1-0.3428)}{1123}} = 0.014164097619600914 \\ \end{align}\]1.4 Std. Error of Difference
\[\begin{align} \text{SE}_{\text{difference}} &= \sqrt{\text{SE}^2_A + \text{SE}^2_B} \\ &= \sqrt{0.013886^2 + 0.014164^2} \\ &= 0.0198356539 \end{align}\]1.5 Confidence Interval for Conversion Rate
만약 population’s standard deviation \(\sigma\) 을 알고 있다면 Z-Value 를 사용하면 됩니다.
또는 일반적으로 계산의 용의성 때문에 Z-value를 사용하기도 합니다. (귀차니즘)
- p : Conversion rate (the mean of the bernoulli distribution)
- n : sample size
- \(\frac{\sigma}{\sqrt{n}}\) : Standard Error \(\sigma_{\bar{x}}\)
- \(Z_{1-\alpha/2}\) : Z value of the standard deviation
- \(Z_{1-\alpha/2} = 1.96\) for 95% confidence ( \(\alpha = 0.05\) )
- \(Z_{1-\alpha/2} = 2.57\) for 99% confidence ( \(\alpha = 0.01\) )
- \(\alpha/2\): 2로 나눠주는 이유는 양측검정(two-tailed test)를 하기 위함이다. 신뢰구간은 +- 양쪽을 모두 본다. 따라서 양측검정이 맞다.
Confidence Level | Python | Python | \(Z\) Value |
---|---|---|---|
80% 신뢰수준 | norm.ppf(1 - (1-0.8)/2) | norm.ppf(0.9) | 1.2815 |
85% 신뢰수준 | norm.ppf(1 - (1-0.85)/2) | norm.ppf(0.925) | 1.4395 |
90% 신뢰수준 | norm.ppf(1 - (1-0.9)/2) | norm.ppf(0.95) | 1.6448 |
95% 신뢰수준 | norm.ppf(1 - (1-0.95)/2) | norm.ppf(0.975) | 1.9599 |
99% 신뢰수준 | norm.ppf(1 - (1-0.99)/2) | norm.ppf(0.995) | 2.5782 |
99.5% 신뢰수준 | norm.ppf(1 - (1-0.995)/2) | norm.ppf(0.9975) | 2.8070 |
99.9% 신뢰수준 | norm.ppf(1 - (1-0.999)/2) | norm.ppf(0.9995) | 3.2905 |
예를 들어서 34.28% conversion rate 그리고 95% 신뢰수준의 confidence interval은 다음과 같이 계산합니다.
\[\begin{align} CL_A &= 0.3428 \pm 1.9599 \sqrt{ \frac{0.3428 ( 1- 0.3428)}{1123} } \\ &= 34.28\% \pm 2.776\% \end{align}\]즉 31.5% ~ 37.05% 사이의 확률값을 갖습니다.
norm.ppf 는 N(0, 1)인 표준정규분포에서 0에서부터 95%까지의 면적에 대한 확률을 norm.ppf(0.975)로 계산할 수 있습니다.
즉 norm.ppf(0.5) = 0 입니다.
1.5.1 My Confidence Interval for Conversion Rate
import numpy as np
from scipy.stats import norm
def standard_error(n, p):
# 베르누이 분포에 대한 standard deviation = sqrt(p(1-p))
return np.sqrt(p * (1 - p)/n)
n_a = 1093
n_b = 1123
p_a = 330/n_a
p_b = 385/n_b
se_a = standard_error(n_a, p_a)
se_b = standard_error(n_b, p_b)
Z = norm.ppf(1 - (1-0.95)/2) # 1.959963984540054 // +- 양측으로 신뢰구간을 찾는 것이기 때문에 나누기2로 양측검정으로 간다
ci_a = Z * se_a
ci_b = Z * se_b
print(f'Confidence Interval for A: {p_a*100:.4} ±{ci_a*100:.4}% | {(p_a-ci_a)*100:.6}% ~ {(p_a+ci_a)*100:.6}%')
print(f'Confidence Interval for B: {p_b*100:.4} ±{ci_b*100:.4}% | {(p_b-ci_b)*100:.6}% ~ {(p_b+ci_b)*100:.6}%')
Confidence Interval for A: 30.19 ±2.722% | 27.4705% ~ 32.9138%
Confidence Interval for B: 34.28 ±2.776% | 31.5071% ~ 37.0593%
1.5.2 Scipy Confidence Interval for Conversion Rate
from scipy.stats import t, norm
def standard_error(n, p):
# 베르누이 분포에 대한 standard deviation = sqrt(p(1-p))
return np.sqrt(p * (1 - p)/n)
p_a = 330/n_a
p_b = 385/n_b
se_a = standard_error(n_a, p_a)
se_b = standard_error(n_b, p_b)
n_interval = norm.interval(0.95, loc=p_a, scale=se_a)
t_interval = t.interval(0.95, n_a, loc=p_a, scale=se_a)
print(f'CI for A with norm | {n_interval[0]*100:.6}% ~ {n_interval[1]*100:.6}%')
print(f'CI for A with t | {t_interval[0]*100:.6}% ~ {t_interval[1]*100:.6}%')
n_interval = norm.interval(0.95, loc=p_b, scale=se_b)
t_interval = t.interval(0.95, n_b, loc=p_b, scale=se_b)
print(f'CI for B with norm | {n_interval[0]*100:.6}% ~ {n_interval[1]*100:.6}%')
print(f'CI for B with t | {t_interval[0]*100:.6}% ~ {t_interval[1]*100:.6}%')
CI for A with norm | 27.4705% ~ 32.9138%
CI for A with t | 27.4674% ~ 32.9168%
CI for B with norm | 31.5071% ~ 37.0593%
CI for B with t | 31.5041% ~ 37.0623%
1.5.2 모표준편차를 모를때.. (T-statistic)
\[\begin{align} CI &= \bar{x} \pm T_{a/2} \frac{s}{\sqrt{n-1}} \\ &= p \pm T_{a/2} \sqrt{ \frac{p(1-p)}{n-1} } \end{align}\]Confidence Level | Python | Python | \(T\) Value (n=1123) |
---|---|---|---|
80% 신뢰수준 | t.ppf(1 - (1-0.8)/2, df=n-1) | t.ppf(0.9, df=n-1) | 1.2823 |
85% 신뢰수준 | t.ppf(1 - (1-0.85)/2, df=n-1) | t.ppf(0.925, df=n-1) | 1.4405 |
90% 신뢰수준 | t.ppf(1 - (1-0.9)/2, df=n-1) | t.ppf(0.95, df=n-1) | 1.6462 |
95% 신뢰수준 | t.ppf(1 - (1-0.95)/2, df=n-1) | t.ppf(0.975, df=n-1) | 1.962 |
99% 신뢰수준 | t.ppf(1 - (1-0.99)/2, df=n-1) | t.ppf(0.995, df=n-1) | 2.5802 |
99.5% 신뢰수준 | t.ppf(1 - (1-0.995)/2, df=n-1) | t.ppf(0.9975, df=n-1) | 2.8125 |
99.9% 신뢰수준 | t.ppf(1 - (1-0.999)/2, df=n-1) | t.ppf(0.9995, df=n-1) | 3.2992 |
예를 들어서 34.28% conversion rate 그리고 95% 신뢰수준의 confidence interval은 다음과 같이 계산합니다.
\[\begin{align} CL_A &= 0.3428 \pm 1.962 \sqrt{ \frac{0.3428 ( 1- 0.3428)}{1123} } \\ &= 34.28\% \pm 2.779\% \end{align}\]보시면 알겠지만.. Z-value 사용했을때와 그닥 많이 차이가 나지 않습니다.
import numpy as np
from scipy.stats import ttest_ind_from_stats
def standard_deviation(n, p):
# 베르누이 분포에 대한 standard deviation = sqrt(p(1-p))
return np.sqrt(p * (1 - p))
n_a = 1093
n_b = 1123
p_a = 330/n_a
p_b = 385/n_b
std_a = standard_deviation(n_a, p_a)
std_b = standard_deviation(n_b, p_b)
t_statistic, t_pvalue = ttest_ind_from_stats(mean1=p_a, std1=std_a, nobs1=n_a,
mean2=p_b, std2=std_b, nobs2=n_b)
print(f't-statistic: {t_statistic:.4}')
print(f'p-value : {t_pvalue/2:.4} for one-tailed test')
print(f'p-value : {t_pvalue:.4} for two-tailed test')
t-statistic: -2.062
p-value : 0.01968 for one-tailed test
p-value : 0.03937 for two-tailed test
1.6 P-Value
1.6.1 P-Value with Z-Test
import numpy as np
from scipy.stats import norm
def standard_deviation(n, p):
# 베르누이 분포에 대한 standard deviation = sqrt(p(1-p))
return np.sqrt(p * (1 - p))
n_a = 1093
n_b = 1123
p_a = 330/n_a
p_b = 385/n_b
std_a = standard_deviation(n_a, p_a)
std_b = standard_deviation(n_b, p_b)
Z = (p_b-p_a)/np.sqrt(std_b**2/n_b + std_a**2/n_a)
p_value = norm.sf(Z)
print(f'Z-Score: {Z}')
print(f'P-Value: {p_value}')
Z-Score: 2.062467084154844
P-Value: 0.019581644108032148
1.6.1 P-Value with T-Test
import numpy as np
from scipy.stats import ttest_ind_from_stats
def standard_deviation(n, p):
# 베르누이 분포에 대한 standard deviation = sqrt(p(1-p))
return np.sqrt(p * (1 - p))
n_a = 1093
n_b = 1123
p_a = 330/n_a
p_b = 385/n_b
std_a = standard_deviation(n_a, p_a)
std_b = standard_deviation(n_b, p_b)
t_statistic, t_pvalue = ttest_ind_from_stats(mean1=p_a, std1=std_a, nobs1=n_a,
mean2=p_b, std2=std_b, nobs2=n_b)
print(f't-statistic: {t_statistic}')
print(f'p-value : {t_pvalue/2}')
t-statistic: -2.061536295011771
p-value : 0.019684200112302923
1.6.1 P-Value with Chi-Squared Test
import pandas as pd
from scipy.stats import chi2_contingency
n_a = 1093
n_b = 1123
p_a = 330/n_a
p_b = 385/n_b
df = pd.DataFrame([[n_a-330, 330], [n_b-385, 385]], columns=['Not Converted', 'Converted'], index=['A', 'B'])
display(df)
chi_statistic, p_value, dof, expected_df = chi2_contingency(df)
expected_df = pd.DataFrame(expected_df, columns=df.columns, index=df.index)
display(expected_df)
print('degree of freedom :', dof)
print('chi square statistic:', chi_statistic)
print('p-value :', p_value)
2. 자세한 설명
2.1 Standard Error
베르누이 분포는 \(P(X=1) = p\) 그리고 \(P(X=0) = (1-p)\) 와 같이 오직 두가지 가능한 결과가 일어난다고 했을때 사용되는 분포입니다.
여기서 p는 variation의 conversion rate를 가르킵니다.
이때 mean, variance 그리고 standard deviation 다음과 같습니다.
Central limit theorem에 따르면 다수의 표본 평균을 계산함으로서 모평균을 추정할 수 있습니다.
즉 표본평균으로 나온 \(p\) 의 분포는 정규분포를 따르며,
standard deviation of the population \(\sigma\)는 표본평균의 표준오차 (the standard error of the sample mean) 와 동일합니다.
The standard deviation of the sample mean 의 공식은 아래와 같습니다. (베르누이 분포에 대해서..)
추가적으로 \(p\) 는 정규분포를 따름으로 다음과 같이 정의할수 있습니다.
\[\hat{p} \sim \mathcal{Normal} \left(u=p, \sigma= \sqrt{\frac{p(1-p)}{n}} \right)\]아주쉽게 말하면, 표본 평균의 standard error는 표본이 따르는 정규분포의 1 standard deviation이라고 말할 수 있습니다.
import numpy as np
from scipy.stats import norm
def standard_error(n, p):
return np.sqrt(p * (1 - p) / n)
n_a = 1093
n_b = 1123
p_a = 330/n_a
p_b = 385/n_b
se_a = standard_error(n_a, p_a)
se_b = standard_error(n_b, p_b)
print(f'[A] n:{n_a} | conversion: {p_a:6.4} | standard error: {se_a:6.4}')
print(f'[B] n:{n_b} | conversion: {p_b:6.4} | standard error: {se_b:6.4}')
# Visualization
rv_a = norm(loc=p_a, scale=se_a)
rv_b = norm(loc=p_b, scale=se_b)
x = np.linspace(0.24, 0.41, 1000)
fig, ax = subplots(figsize=(8, 5))
sns.lineplot(x, rv_a.pdf(x), color='red', label='A')
sns.lineplot(x, rv_b.pdf(x), color='blue', label='B')
ax.axvline(x=p_a, c='red', alpha=0.5, linestyle='--')
ax.axvline(x=p_b, c='blue', alpha=0.5, linestyle='--')
plt.ylabel('PDF')
plt.grid()
위의 그래프를 보면, 두 그룹간에 서로 겹치지 않고 꽤 떨어져 있음을 볼 수 있습니다.
만약 A, B둘다 귀무가설대로 차이가 없다면 두 분포는 꽤나 겹치는 부분이 많습니다.
2.2 Z-Score & Z Table
population mean (모평균) 그리고 population standard deviation (모표준편차)을 알고 있으며 sample size가 30개 이상인 경우에는 standard score는 다음과 같이 계산합니다.
\[z = \frac{x - \mu}{\sigma}\]- \(\mu\) : the mean of the population
- \(\sigma\) : the standard devistion of the population
- \(z\): 모평균에서 표준편차로 얼마나 떨어져 있는지를 나타내며, negative라면 평균보다 작고, positive이면 평균보다 크다는 의미
현실적으로 population mean 그리고 population standard deviation을 알고 있는 경우는 특이 많이 없습니다.
이 경우 sample mean 그리고 sample standard deviation을 사용해서 standard score를 계산할 수 있습니다.
- \(\bar{x}\) : the mean of the sample
- \(s\) : the standard deviationn of the sample
Z-Score를 계산했다면, unit normal distribution(mean=0, std=1)에서 해당 Z-Score까지의 면적에 대한 확률을 표준정규분포표를 통해서 확인을 할 수 있습니다.
표준정규분포표는 표준정규분포(mean=0, std=1)에서 0에서부터 Z까지의 확률을 나타낸 것입니다.
2.3 Bernoulli Distribution
Population이 \(X_i^{iid} \sim Bernoulli(p)\) 를 따를때 위에서 언급했듯이 mean, variance, standard deviation은 다음과 같습니다.
- mean: \(\mu = E[X] = p\)
- variance: \(\sigma^2 = Var(X) = p(1-p)\)
- std: \(\sigma = Std(X) = \sqrt{p(1-p)}\)
- \(p\) : \(x \in [1, 0]\) 을 따를때 1일 확률. 즉 1을 선택할 확률 <- 우리가 알아내고자 하는 estimator값
- 더 쉽게 이야기 하면 p는 conversion rate이다
Central Limit Theorem에 따르면 the mean of the sample means 그리고 the standard deviation of the sample means는 다음과 같습니다.
\(\begin{align} \text{the mean of the sample means } &= \mu_{\bar{x}} = \mu = p \\ \text{the standard deviation of the sample means} &= \sigma_{\bar{x}} = \frac{s}{\sqrt{n}} = \sqrt{\frac{p(1-p)}{n}} \end{align}\)
- 쉽게 말해 표본평균의 평균은 모평균을 따른다
- 표본평균 \(p\) 의 분포는 정규분포를 따른다 (central theorem)
- standard deviation \(\sigma\) 는 standard error of the mean (standard error)과 동일하다
따라서 다음과 같이 정의할 수 있습니다.
\[\hat{p} \sim \mathscr{N} \left(\mu=p, \sigma= \sqrt{\frac{p(1-p)}{n}} \right)\]2.4 Confidence Interval
목표는 표본평균값이 \(p\) 모평균 \(\mu\) 안에 95%의 확률로 confidence interval안에 포함되는 것을 구하고자 합니다.
즉 100번의 표본 평균을 구했을때 해당 평균이 95번 신뢰구간안에 포함되는 확률을 구하고자 합니다.
- \(p\) : sample mean
- \(SE\) : standard error
- \(1.96\) : critical value for 95% confidence level
즉 평균값 \(p\) 를 중심으로 플러스 마이너스 표준편차 * critical value (1.96)을 함으로서 95%안에 들어올 구간을 정하는 것입니다.
Bernoulli distribution에 대한 Confidence Interval의 공식은 다음과 같습니다.
Confidence interval을 계산하기 전에 먼저 confidence coefficient \(Z_{\alpha/2}\) (신뢰계수) 를 계산해야 합니다.
- \(\alpha\) : significance level (유의수준) -> 0.05 같은 값을 사용 (H0의 확률)
- \(\alpha/2\) : 정규분포의 양쪽에서 유의수준을 보기 때문에 2로 나눈다
- \(Z_{\alpha/2}\) : 임계값. 예를 들어 95% 신뢰도는 \(1 - \alpha = 0.95\ (\alpha = 0.05)\) 이고, 2로 나누면 \((1 - \alpha)/2 = 0.475\) 가 됩니다.
표준정규분포에서 47.5%의 확률에 해당하는 z-value는 1.96을 확인 할 수 있습니다.
즉 0에서 시작해서 우측으로 47.5% 면적에 해당하는 확률의 z-value가 1.96이라는 뜻입니다.
공식에 대한 유도는 다음과 같습니다.
따라서 샘플 평균 \(\bar{x}\) 에 대한 \(100 (1 - \alpha)\) % confidence interval 은 다음과 같습니다.
\[\begin{align} \text{confidence interval} &= p \pm Z_{\alpha/2} \cdot \frac{s}{\sqrt{n}} \\ &= p \pm Z_{\alpha/2} \cdot \sqrt{\frac{p(1-p)}{n}} \end{align}\]- 95% Confidence Interval ( \(\alpha = 0.05\) ) : \(Z_{\alpha/2} = Z_{0.025} = 1.96\)
- 99% Confidence Interval ( \(\alpha = 0.01\) ) : \(Z_{\alpha/2} = Z_{0.005} = 2.575\)
from scipy.stats import t, norm
p_a = 330/n_a
p_b = 385/n_b
n_interval = norm.interval(0.95, loc=p_a, scale=se_a)
t_interval = t.interval(0.95, n_a, loc=p_a, scale=se_a)
print(f'CI with norm | left: {n_interval[0]:.4} right: {n_interval[1]:.4}')
print(f'CI with t | left: {t_interval[0]:.4} right: {t_interval[1]:.4}')
# CI with norm | left: 0.2747 right: 0.3291
# CI with t | left: 0.2747 right: 0.3292
def standard_error(n, p):
return np.sqrt(p * (1 - p) / n)
def confidence_interval(n, p, alpha=0.05):
se = standard_error(n, p)
margin_error = norm.ppf(1-alpha/2) * se
return margin_error
z_a = confidence_interval(n_a, p_a)
z_b = confidence_interval(n_b, p_b)
print('[A]')
print('95% confidence level | Z:', norm.ppf(1-0.05/2))
print(f'CI with norm: z-value: {z_a:.4} | left: {p_a - z_a:.4} | right: +{p_a + z_a:.4}')
print(f'confidence interval: {p_a*100:5.4}% +-{z_a*100:<.4}%')
print('\n[B]')
print('95% confidence level | Z:', norm.ppf(1-0.05/2))
print(f'CI with norm: z-value: {z_b:.4} | left: {p_b - z_b:.4} | right: +{p_b + z_b:.4}')
print(f'confidence interval: {p_b*100:5.4}% +-{z_b*100:<.4}%')
[A]
95% confidence level | Z: 1.959963984540054
CI with norm: z-value: 0.02722 | left: 0.2747 | right: +0.3291
confidence interval: 30.19% +-2.722%
[B]
95% confidence level | Z: 1.959963984540054
CI with norm: z-value: 0.02776 | left: 0.3151 | right: +0.3706
confidence interval: 34.28% +-2.776%
Basic Math for Bernoulli Distribution
Variance Ruls
\(Var(X + Y) = Var(X) + Var(Y) = \sigma^2_X + \sigma^2_Y\)
\(Var(X - Y) = Var(X) + Var(Y) = \sigma^2_X + \sigma^2_Y\)
두 분포간의 차이
두 분포간의 차이는 두 분포의 평균값의 거리라고 보면 됩니다.
\(\hat{d} = p_b - p_a\)
따라서 the difference of the two normal distributions의 variacne 그리고 standard deviation은 다음과 같습니다.
\[\begin{align} Var(\hat{d}) &= Var(p_b - p_a) \\ &= Var(p_a) + Var(p_b) \\ &= \frac{s^2_a}{n} + \frac{s^2_b}{n} \\ &= \frac{p_a(1-p_a)}{n_a} + \frac{p_b(1-p_b)}{n_b} \end{align}\] \[\begin{align} Std(\hat{d}) &= \sqrt{Var(d)} \\ &= \sqrt{\frac{s^2_a}{n_a} + \frac{s^2_b}{n_b}} \\ &= \sqrt{\frac{p_a(1-p_a)}{n_a} + \frac{p_b(1-p_b)}{n_b}} \end{align}\]2.5 Basic Math for Bernoulli Distribution
Variance Ruls
\(Var(X + Y) = Var(X) + Var(Y) = \sigma^2_X + \sigma^2_Y\)
\(Var(X - Y) = Var(X) + Var(Y) = \sigma^2_X + \sigma^2_Y\)
두 분포간의 차이
두 분포간의 차이는 두 분포의 평균값의 거리라고 보면 됩니다.
\(\hat{d} = p_b - p_a\)
따라서 the difference of the two normal distributions의 variacne 그리고 standard deviation은 다음과 같습니다.
\[\begin{align} Var(\hat{d}) &= Var(p_b - p_a) \\ &= Var(p_a) + Var(p_b) \\ &= \frac{s^2_a}{n} + \frac{s^2_b}{n} \\ &= \frac{p_a(1-p_a)}{n_a} + \frac{p_b(1-p_b)}{n_b} \end{align}\] \[\begin{align} Std(\hat{d}) &= \sqrt{Var(d)} \\ &= \sqrt{\frac{s^2_a}{n_a} + \frac{s^2_b}{n_b}} \\ &= \sqrt{\frac{p_a(1-p_a)}{n_a} + \frac{p_b(1-p_b)}{n_b}} \end{align}\]2.6 Satterwaite Approximation and Pooled Standard Error
\[\begin{align} \text{satterwaite} &= \sqrt{\frac{s^2_a}{n_a} + \frac{s^2_b}{n_b}} \\ \text{pooled standard error} &= Sp \sqrt{\frac{1}{n_a} + \frac{1}{n_b}} \end{align}\]- 새터스웨이트는 독립표본 검정 (두 집단 간에 평균이 차이가 있는지 검증하는 분석)으로서 모분산이 같지 않을 경우에 사용합니다.
- Pooled Standard Error는 사실상 satterwaite와 동일하다고 보면 됩니다. 다만 pooled standard error의 경우 두 분포는 동일하다고 가정을 합니다.
- \(s^2\) : standard deviation from the sample. \(s^2\) 는 variance.
Pooled standard error를 통해서 두 그룹간의 standard deviation을 계산한다면 다음과 같습니다.
\[\begin{align} Std(\hat{d}) &= \sqrt{Var(\hat{d})} &[1] \\ &= \sqrt{\frac{s^2_p}{n_a} + \frac{s^2_p}{n_b}} & [2] \\ &= \sqrt{s^2_p \left( \frac{1}{n_a} + \frac{1}{n_b} \right)} &[3] \\ &= \sqrt{ \hat{P}_p \left(1-\hat{P}_p\right) \left( \frac{1}{n_a} + \frac{1}{n_b} \right)} &[4] \\ \end{align}\] \[\hat{P}_p = \frac{p_a n_a + p_b n_b}{n_a + n_b}\]